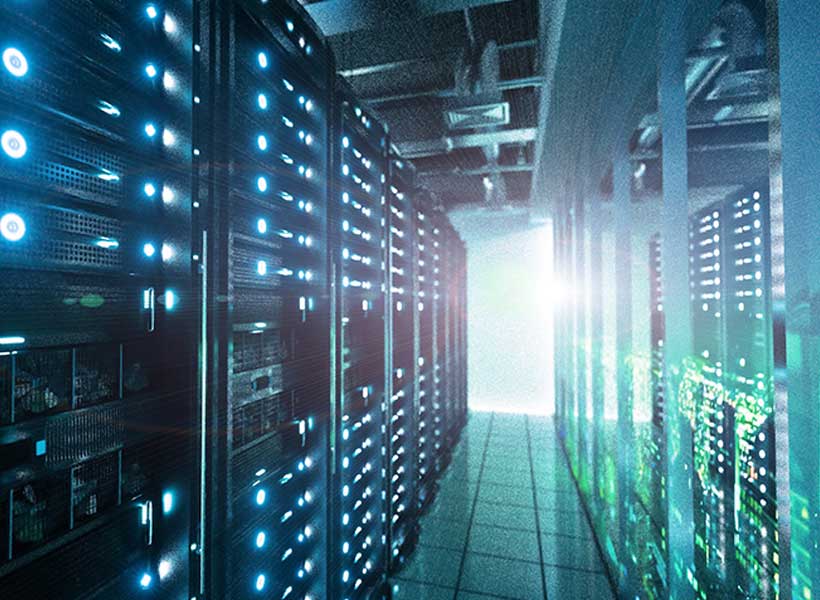
The rise of quantum computing is more than a technological advancement; it marks a profound shift in the world of cybersecurity, especially when considering the actions of state-sponsored cyber actors. Quantum technology has the power to upend the very foundations of digital security, promising to dismantle current encryption standards, enhance offensive capabilities, and recalibrate the balance of cyber power globally. As leading nations like China, Russia, and others intensify their investments in quantum research, the potential repercussions for cybersecurity and international relations are becoming alarmingly clear.
Imagine a world where encrypted communications, long thought to be secure, could be broken in mere seconds. Today, encryption standards such as RSA or ECC rely on complex mathematical problems that would take traditional computers thousands of years to solve. Quantum computing, however, changes this equation. Using quantum algorithms like Shor’s, a sufficiently powerful quantum computer could factorize these massive numbers, effectively rendering these encryption methods obsolete.
This capability could give state actors the ability to decrypt communications, access sensitive governmental data, and breach secure systems in real time, transforming cyber espionage. Instead of months spent infiltrating networks and monitoring data flow, quantum computing could provide immediate access to critical information, bypassing traditional defenses entirely.