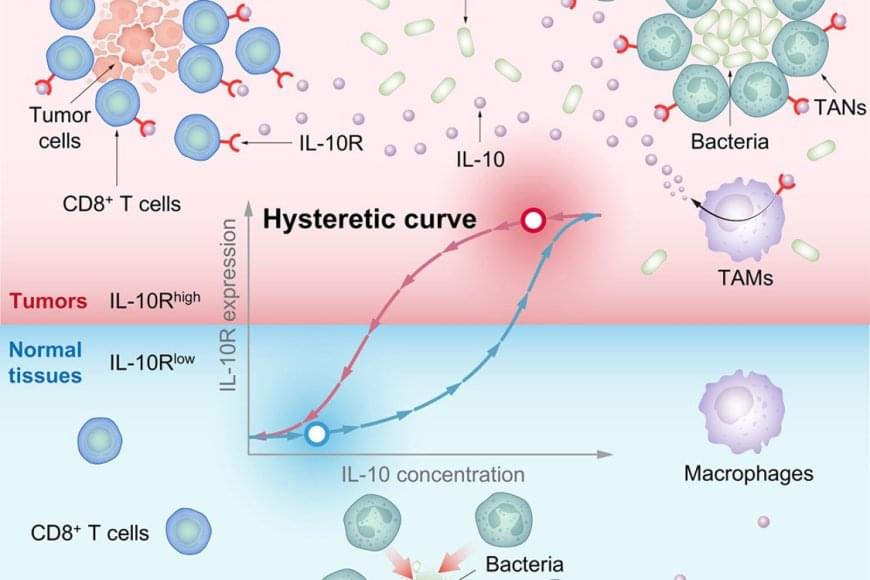
In this study, researchers engineered an attenuated strain, Designer Bacteria 1 (DB1), which efficiently survives and proliferates in tumor tissues while being cleared in normal tissues, achieving a remarkable “tumor-targeting” effect as well as “tumor-clearing” effect.
To understand how DB1 simultaneously achieves these effects, researchers investigated the interactions between the bacteria and tumors. They discovered that DB1’s antitumor efficacy is closely linked to tissue-resident memory (TRM) CD8+ T cells within the tumor, which are reinvigorated and expanded following DB1 therapy. Interleukin-10 (IL-10) plays a crucial role in mediating this effect, with efficacy depending on the high expression of interleukin-10 receptor (IL-10R) on CD8+ TRM cells.
To investigate the molecular mechanisms underlying the high expression of IL-10R on CD8+ TRM cells, researchers conducted a series of computational and quantitative experiments. They found that IL-10 binds to IL-10R on CD8+ TRM cells, activating the STAT3 protein and further promoting IL-10R expression. This established a positive feedback loop, enabling cells to bind more IL-10 and creating a nonlinear hysteretic effect, whereby CD8+ TRM cells “memorize” previous IL-10 stimulation during tumorigenesis. The high expression of IL-10R on CD8+ TRM cells was exploited by a bacteria-induced IL-10 surge, which activated and expanded CD8+ TRM cells to clear tumor cells.
To examine the source of IL-10 within the tumor microenvironment (TME) after bacterial therapy, researchers found that tumor-associated macrophages (TAMs) upregulate IL-10 expression following DB1 stimulation via the Toll-like Receptor 4 (TLR4) signaling pathway. Interestingly, IL-10 reduced the migration speed of tumor-associated neutrophils (TANs), aiding DB1 in evading rapid clearance. These processes depended on high IL-10R expression in tumor-associated immune cells, highlighting the critical role of IL-10R hysteresis.
A research team elucidated the mechanism behind bacterial cancer therapy using a genetically engineered bacterial strain. Their findings were published in Cell.
Exploring the use of antitumor bacteria in cancer therapy dates back to the 1860s. Despite this long history, however, clinical application of bacterial-based cancer therapy has faced significant challenges in terms of safety and efficacy.