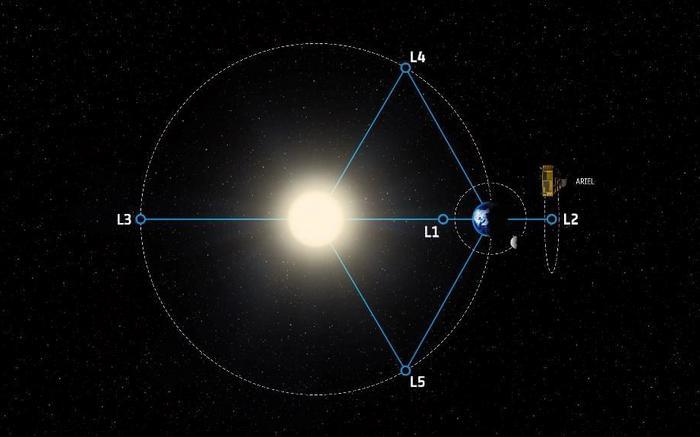
Can machine learning be used to advance exoplanet science, and can this be done by non-scientists, as well? This is what Ariel Data Challenge 2024 hopes to address as participants from around the world will compete to develop machine learning algorithms designed to analyze data from space telescopes with the goal of gaining greater insight into exoplanet atmospheres. This competition will be featured at the NeurIPS 2024 machine learning conference and holds the potential to not only advance the field of exoplanets but also enable non-scientists to conduct pioneering research, as well.
“By supporting this challenge, we aim to find new ways of using AI and machine learning to develop our understanding of the universe,” said Dr. Caroline Harper, who is the Head of Space Science at the UK Space Agency. “Exoplanets are likely to be more numerous in our galaxy than the stars themselves and the techniques developed through this prestigious competition could help open new windows for us to learn about the composition of their atmospheres, and even their weather.”
Along with the UK Space Agency, other institutions supporting this challenge include the STFC DiRAC HPC Facility, European Space Agency (ESA), and STFC RAL Space. The competition is named after the ESA’s Ariel Space Mission, which is currently scheduled for launch in 2029 with the goal of using the transit method for identifying more than 1,000 exoplanets.