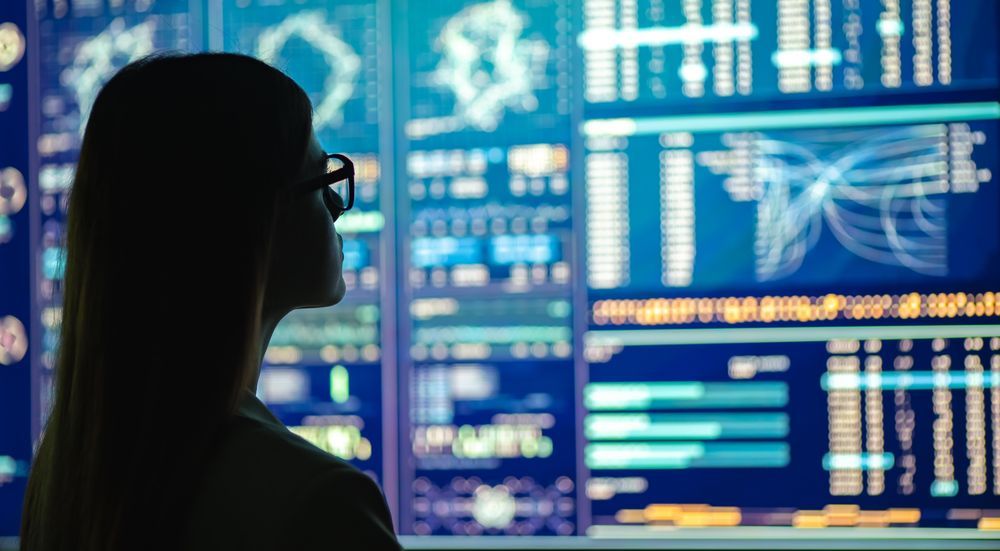
The hidden secret of artificial intelligence is that much of it is actually powered by humans. Well, to be specific, the supervised learning algorithms that have gained much of the attention recently are dependent on humans to provide well-labeled training data that can be used to train machine learning algorithms. Since machines have to first be taught, they can’t teach themselves (yet), so it falls upon the capabilities of humans to do this training. This is the secret achilles heel of AI: the need for humans to teach machines the things that they are not yet able to do on their own.
Machine learning is what powers today’s AI systems. Organizations are implementing one or more of the seven patterns of AI, including computer vision, natural language processing, predictive analytics, autonomous systems, pattern and anomaly detection, goal-driven systems, and hyperpersonalization across a wide range of applications. However, in order for these systems to be able to create accurate generalizations, these machine learning systems must be trained on data. The more advanced forms of machine learning, especially deep learning neural networks, require significant volumes of data to be able to create models with desired levels of accuracy. It goes without saying then, that the machine learning data needs to be clean, accurate, complete, and well-labeled so the resulting machine learning models are accurate. Whereas it has always been the case that garbage in is garbage out in computing, it is especially the case with regards to machine learning data.
According to analyst firm Cognilytica, over 80% of AI project time is spent preparing and labeling data for use in machine learning projects: